So awhile ago I mentioned that I was trying to add a new shared-memory cache for the next version of the KDE platform. It’s almost done now, and has been submitted for review (both old-skool on kde-core-devel, and all Web 2.0-style on our review board).
Given the number of things I had to think about while implementing it (and I promise you that even still it’s not fully as thought-out as it could be), I decided that I could probably make a half-decent, if very technical series of posts about the implementation process.
I’ve got a basic outline set out, but without further ado, I’ll go over in this post what exactly a shared-memory cache is, why KDE has one now, and why I’m trying to make a different one.
Why a shared-memory cache?
Most of the programmer types are already familiar with the idea of a cache: You have some input that isn’t particularly helpful right now, you have a function to turn that non-helpful input into something you can use, but that function takes awhile to run. So, you save the output of that function (the value) once, somewhere where you can refer to it later (by a key) if you need it. The idea is to trade some extra memory usage for reduced time requirements. Caching is used everywhere, in your CPU, in your Web browser, the operating system, and more.
Now, the shared-memory part allows this cache to be shared between running processes (if you don’t know what a process is, just think of it as an instance of a running program. Each different instance, even of the same program, would be a different process). The operating system normally does a very good job of making sure that different processes can’t interfere with each other, but there are times when it makes sense to open a couple of gateways between them to let them share the burden. In KDE’s case, many of the icons used by a standard KDE application will be used unchanged by other KDE programs, so it makes sense for us to cache generated icons for use by other KDE program. We could simply write the icons out to disk where other programs could read them, but putting them into shared memory allows for the near-immediate transfer of that data without any disk I/O.
I’d like to find examples of current shared-memory caches (besides our current KPixmapCache), but the only ones I can find are the fully distributed type like memcached. Cairo has a cache for glyphs, but that seems to be done per-process. GTK+ has a cache which is designed to be read in directly using mmap(2), but not necessarily to be accessed via shared memory. Let me know if you find any though!
So again, in our case we use a shared-memory cache in large part to handle icon loading and Plasma themes (both potentially heavy users of SVG icons). This gives us two speedups: 1) We don’t always have to re-generate a pixmap from the source data (a very big speedup when the source is an SVG), and 2) If one process generates a pixmap, every other KDE process can get access to the results nearly instantly.
What KDE currently does
My interest in shared-memory caching came about from looking into some bugs reported against our current cache, KPixmapCache, which was developed in conjunction with the lead-up to KDE 4.0 to allow the new Plasma subsystem and the existing icon subsystem to use the fancy SVG graphics without taking forever.
In addition, KPixmapCache had a feature where not only could it cache the image data (the 0’s and 1’s that make up the image itself), but also the resulting QPixmap handle to the image as it exists in “the graphics memory” (I’ll gloss over the distinction for now, it will be important in a future part).
KPixmapCache is implemented by hosting two different files in a known location, one ending in .index and the other ending in .data. Respectively these files hold the index metadata needed for the cache to work, and the actual image data.
Anytime you talk about shared resources, you also need to think about how to protect access to those shared resources to keep everything consistent. KPixmapCache uses the trusty KLockFile to protect against concurrent access (this has the benefit of being safe if the partition is mounted on NFS, although I think the reason is more because that’s what already existed in kdelibs).
From there, KPixmapCache uses a sorted binary tree (sorted by the hash code) to manage the index, and treats the .data file as a simple block of memory. Every time a new item is entered into the cache, a new chunk of free space is allocated from the .data file, even if there already exists empty space. Likewise, if a new index entry is required to insert an item, it is always added at the end of the index (with one exception, when overwriting an existing index entry due to a hash collision). I said the index was sorted earlier, but that might seem incompatible with always adding new entries at the end. What the implementer did to solve this problem is hold pointers to the actual children and parent in each index item, that way a virtual hierarchy could be arranged. The binary tree is never re-arranged (like in AVL trees or red-black trees), so the initial root node is always the root node. The overall structure looks like this figure:
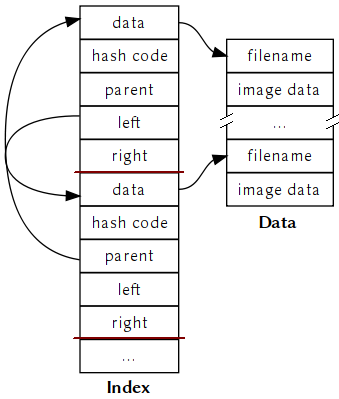
One disadvantage to this architecture is that it is difficult and inefficient to delete entries that should be expired out of the cache. Properly removing an entry would require possibly having to move entries in the data file in order to minimize fragmentation. This alone wouldn’t be a large deal (I end up having to do the same thing in KSharedDataCache), but updating the .index file is even harder, since it requires updating information for both parent and children (although again, not impossible by any means). Avoiding fragmentation in the index would require either moving nodes around in the index file (possibly recursively), or having to scan for the first free node when adding items. None of these are big problems, but it does make the implementation more annoying.
KPixmapCache worked around all of this by punting the problem: No entries ever got removed until the cache filled up. At this point the entries would be ranked by whatever the expiration policy in effect was (i.e. least recently used preferred, newest preferred, etc.), a new (smaller) cache would be constructed holding the most-desired entries, and the old cache would be deleted. Although infrequent, this could possibly take a not-insignificant amount of time when it did happen.
So why a new implementation?
Probably the one thing that led to me starting from a different architecture however, was the interface to KPixmapCache: It is designed to be subclassed, and to allow subclasses access to both the index and individual data items through a QDataStream (see KIconCache for an example usage). Doing this meant the internal code had to use a QIODevice to interface to the data and index, and so what ends up happening is that even though KPixmapCache tries to place all of the data in shared memory, it always ends up accessing it like it was a disk file anyways (even though a memory-to-QIODevice adapter is used).
Having to support subclassing (in the public API no less) makes changing many of the implementation details a journey fraught with hazard, and it’s bad enough that any little problem in KPixmapCache seemingly guarantees a crash. Since KPixmapCache is used in very core desktop platform code (Plasma and KIconLoader) I knew I wanted to go a different direction.
So, I started work on a “KIconCache”. However all the work I was doing was hardly specific to icons, and when I’d heard of a developer that was abusing KPixmapCache to hold non-image-data somehow, I decided to make a generic shared-memory cache, KSharedDataCache. Next post I’ll try to explain the direction I decided to take with KSharedDataCache.